Machine learning
How AI is revolutionizing medicine
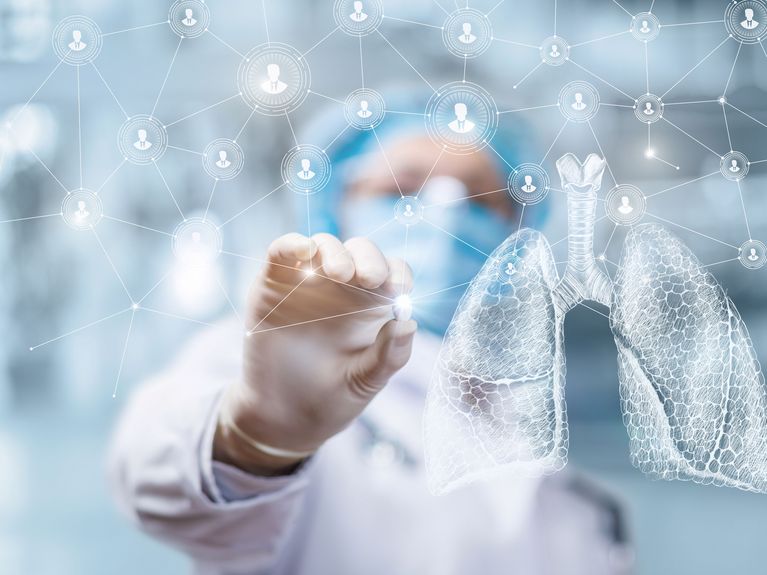
Picture: 2020 Natali _ Mis/Shutterstock.
Medicine is on the verge of another revolution: Artificial intelligence supports doctors in analyzing X-ray and ultrasound images and in improving diagnostics and treatment. Researchers in Germany are also successfully working on novel solutions, and good access to data is important in the process.
This is the story of an unusual treasure hunt. The treasure is not to be found in the forest, but among all the data that constantly accumulates in the healthcare system: Blood pressure measurements, blood values, oxygen saturation, for example, or blood cells examined under the microscope, ultrasound and MRI scans. Unlike a normal treasure hunt, the value only becomes apparent when as much data as possible is examined in detail. The treasure consists of new information captured by computer programs that learn on their own and become better and better. The use of artificial intelligence in medicine will be one of the dominant giant topics in health research over the next few years. Where are we and what are the prospects?
The computer can learn “supervised”
We speak of artificial intelligence when computer programs are capable of learning. And there are two main ways for this learning to take place. One way can be called "supervised learning." Put simply, researchers show the computer a great many similar things, teaching it what is right or wrong, healthy or pathological, a certain cell formation or just not the desired cell formation, depending on the question. The idea behind this: Once the computer has received enough input, it can make the distinction itself. In this way, it can not only relieve doctors of a lot of work, it can also improve the quality of diagnosis and treatment.
A program measures the placenta in pregnant women
Predicting cell fates
Julia Schnabel is Professor of Computational Imaging and AI in Medicine at the Technical University of Munich and Director of the Institute for Computational Imaging and AI in Medicine at Helmholtz Munich, Photo: private
Julia Schnabel, Professor of Computational Imaging and AI in Medicine at the Technical University of Munich and Director of the Institute for Computational Imaging and AI in Medicine at Helmholtz Munich, is taking this approach with her research groups. She oversees not just one particular project, but several. "And there could be even more applications if we had the relevant data in sufficient quantities," says Julia Schnabel. Among other things, she and her team are currently training an algorithm to measure the placenta in pregnant women. The placenta is the mother's organ that supplies the fetus with nourishment. If the placenta is too small, the unborn will be undersupplied. If the pregnancy is far enough along, it may be necessary to deliver the child earlier to prevent it from developing permanent damage. Because the size of the placenta is difficult to determine, doctors currently pay most attention to the growth of the fetus during checkups, and thus, usually notice an undersupply relatively late.
"We have built a construction with several ultrasound heads that should allow doctors to measure the volume of the placenta with the help of the computer," explains Julia Schnabel. The computer can store images from the different transducers almost simultaneously and then use them to determine the volume of the placenta. The prerequisite for this is that the computer recognizes the placenta and its outline. "For this, the computer needs a great many ultrasound images in which the placenta is marked. Fortunately, data from ultrasound images are easy to obtain: It is not associated with risks, nor with circumstances for the patients," says Schnabel.
The computer as a sparring partner for the doctor
The approach worked: The computer program not only measures the size of the placenta quite well. "We fed our algorithm with additional data so that it also recognizes organs and body parts of the fetus. The program can already provide assistance for the doctors at the ultrasound head, for example, when it comes to sounding the baby," Schnabel explains. The goal is not to replace the experts, but to support them: "When it comes to assessing whether a child should be delivered earlier or not, a doctor will always make the decision in the future. However, thanks to computer algorithms, experts will have a better basis for his or her decision in the future. In this way, a clever artificial intelligence could become a kind of sparring partner for the experts in some areas in the future.
The placenta measurement by Schnabel's team is a typical example of how artificial intelligence could advance medicine, and in some areas downright revolutionize it: The computer is fed data, learns, and delivers insights that bring new knowledge to people.
Digital blood analysis
Physicist Carsten Marr wants to better understand how "supervised learning" works. Image: Helmholtz Munich
Physicist Carsten Marr from Helmholtz Munich and his colleagues are taking a similar approach. The researchers have chosen something for which physicians around the world spend hours and days at the microscope: When blood cancer is suspected, several hundred cells in a so-called blood smear are examined and classified by histologists. The results show whether and to what extent the patient is actually suffering from a similar disease, for example, blood cancer, and how a possible treatment can proceed.
Marr and his team fed a so-called neural network with hundreds of thousands of images of individual white blood cells and the information of the cell type. And with success; the cell type predictions Marr's program makes on new images are as good as the histologists' results. "A neural network is a kind of black box. It learns and can then classify new cells itself, but we don't know in detail what criteria it uses to decide," Marr says. That's why he is currently working to better understand these criteria: "The criteria can not only be helpful for histologists. It is also important for us to understand the decision-making process in order to avoid possible mistakes and learning in the wrong direction," Marr adds.
The physicist explains this with an example: "If I want to teach a computer program to distinguish a dog from a wolf and show it countless pictures, it may learn that the wolf is always pictured in the forest, while the dog is seen in rooms and well-tended gardens." If the program decides based on these criteria, it will make wrong decisions, such as classifying a dog in the forest as a wolf. "In medicine in particular, it's about using this black box of machine learning, but also understanding what makes it tick," Marr explains. Only then could computers become trustworthy assistants for physicians.
“Unsupervised” learning
Fabian Theis, director of the Institute of Computational Biology at Helmholtz Munich. Image: Helmholtz Munich
But there is another way to apply artificial intelligence. This path can be called "unsupervised learning," and it is being pursued by Fabian Theis, director of the Institute of Computational Biology at Helmholtz Munich, among others. In contrast to "supervised learning", the computer receives as little assistance as possible in "unsupervised learning". Fabian Theis is working on what is known as single-cell analysis: In this process, the computer receives data about individual cells and their metabolism in each case, and it has to find patterns in them. "We don't tell the computer what is right or wrong, we just give it some additional information. Ideally, it finds out what commonalities there are in certain properties," Theis explains.
In practice, this could mean in the future, for example, that the computer compares the metabolism of cells in the pancreas of people with diabetes with the metabolism of cells in the pancreas of healthy people, and finds out what is different about the defective cells of the diabetes patients. In the next step, specific drugs can then be sought in the future to repair the corresponding cell metabolism changes.
A good 250 kilometers away from Theis, Lena Maier-Hein, a professor of computational medical interventions with a focus on surgical data science and computational biophotonics at the German Cancer Research Center (DKFZ), is researching other applications of artificial intelligence. Among other things, the AI expert is trying to use computers to measure the blood flow to tissue, characterize and differentiate it based on special spectral camera data. Thanks to technology, this can be done much more precisely than the human eye could ever do. The results will contribute to a significant improvement in cancer diagnostics in the future. The computer scientist sees a lot of potential in applying artificial intelligence not only to image interpretation, but also to decision-making. Surgical interventions, for example, could soon be optimized with the help of AI: While today an interdisciplinary team of experts decides how to treat patients based on individual experience, in the near future, experience from past surgeries may be incorporated into decision-making.
"In surgery, there are millions of completed cases in hospitals. With an evaluation of the cases via artificial intelligence, we can share, evaluate and use the experience from these treatments in the future to treat patients in the best possible way," says Lena Maier-Hein. The best example of initial breakthroughs in medicine-based AI is image analysis. In large cancer treatment centers, radiation therapists are already using algorithms to mark tumor tissue. Lena Maier-Hein is familiar with the field because the Medical Image Processing department at DKFZ is headed by her husband, Klaus Maier-Hein. He succeeded in developing a versatile algorithm for analyzing images using AI. Klaus Maier-Hein's advantage: Immense amounts of data from existing imaging have already been available for his research for years. In surgery, on the other hand, Lena Maier-Hein still lacks data; this is usually not recorded before, during and after surgery, or at least not permanently stored. Even when information is available, it is often unusable due to bureaucratic hurdles. Since data is a crucial factor for her research, Lena Maier-Hein has an idea: "Analogous to an organ donor card, one could introduce a data donor card for patient data. In this way, every patient could ultimately help revolutionize surgery using artificial intelligence."
The problem: The healthcare system in Germany is enormously fragmented
One thing is clear: In order to achieve breakthroughs, research requires enormous amounts of data. And this data is not always available in Germany, as Julia Schnabel also reports: "Ultrasound images of the placenta can still be obtained reasonably well; doctors ask patients for their consent and record the ultrasound examination. For much other data, however, it's difficult to get the information in a consistent, comparable format in Germany." That's mainly because the health care system in this country is enormously fragmented. Each state has its own health ministry, and each hospital collects data in its own way. "The healthcare system and also the handling of data in Germany is divided into many segments. That makes a lot of things more difficult," Schnabel says.
The computer scientist returned to Germany from a professional research stay in London just a few months ago: "In the U.K., people have great trust in the National Health Service (NHS), and it is widely believed that the NHS handles health data sensitively."
Germany lacks confidence in data protection
In Germany, on the other hand, there is a real obsession with data protection, says Carsten Marr. "What amazes me is the discrepancy: Everyone throws personal information around on social networks, but when it comes to medical data that is supposed to be used for research, you have to overcome countless hurdles," says Marr. He himself considers data protection enormously important, but this alone rarely stands in the way of research, he says: "Researchers aren't interested in the names, but in the information behind them." Nevertheless, he and his colleagues experience time and again that it is very difficult to obtain medical data for research, if it is available at all.
This is not just about using data for artificial intelligence, but for using data for the rest of medical research. For studies on COVID-19 drugs, for example, Germany is hardly represented, he said, because approvals usually have a long lead time.
In other countries, this is apparently easier, and that is also due to the attitude of the citizens: In Great Britain, people have a great deal of trust in the National Health Service, or NHS, and it is generally assumed that the NHS handles health data sensitively, Julia Schnabel reports. The situation is similar in Denmark, where health data has been stored electronically for years, while in this country there is still a struggle over the health card.
But progress is slowly being made: Last May, the Digitization Act was launched, easing the data protection burden on the medical sector, leaving data secure while also making it more accessible.
New AI Health projects are being set up in Munich, Heidelberg and Karlsruhe
On the research side, there are also upgrades underway: At Helmholtz Munich, the Helmholtz Computational Health Center is currently being established, where Fabian Theis, among others, is conducting research. In Karlsruhe and Heidelberg, the Helmholtz Information & Data Science School for Health (HIDSS4Health) is being established and expanded: "We are supporting 280 doctoral students with the Helmholtz Information & Data Science Academy. This is currently the largest funding program for the digital sciences and a real house number that acts like a magnet for many talented people," says Andreas Kosmider, who is overseeing the expansion of this Germany-wide network as the Helmholtz Association's Head of Strategic Initiatives.
And if there isn't enough data available in this country for the scientists to conduct research with? For Fabian Theis, that's not an obstacle: "At the moment, you have to be pragmatic; especially in collaborations with foreign research institutions, the data often comes from there, too," says Theis. But he is optimistic that there will also be a jolt forward in Germany in terms of data provision. "I consult on the topic in numerous political committees and I see a real will for progress there," says Theis. He adds that the federal structures cannot be fundamentally changed, but at least everything can be coordinated in such a way that new and accessible data is obtained. That would be a win-win-win situation: For research, for patients, and for Germany as a research location.
[Translate to Englisch:]
[Translate to Englisch:]
Podcast-Tipp: "Swarm-Learning in Pandemie-Zeiten" - mit Joachim Schultze vom Deutschen Zentrum für Neurodegenerative Erkrankungen, der sich mit der Frage beschäftigt, wie man große, medizinische Datenmengen möglichst effizient von einer KI auswerten lassen kann.
Readers comments