Foundation Models
New horizons for science
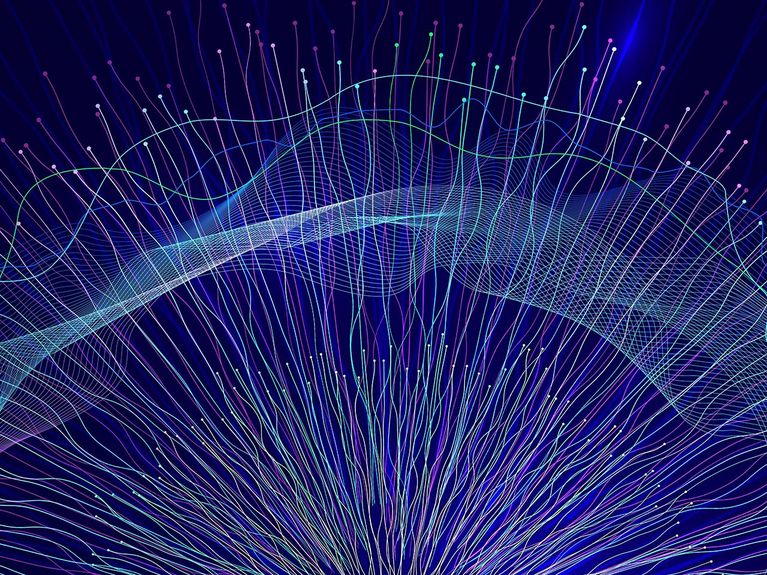
Picture: LariBat/Shutterstock
Foundation models are complex AI applications whose potential for science can hardly be overestimated. They require huge amounts of data, enormous computing power and expertise. The Helmholtz Association is a pioneer in this field.
When it snows deep below the surface of the water, it is of enormous importance for global material cycles. Dead or dying organisms and their faeces that sink to the bottom of the ocean transport huge amounts of carbon and nitrogen. As these flocs continue to sink, they bind carbon that is not released into the atmosphere as carbon dioxide. Without this biological carbon pump, a stable climate on Earth would be unthinkable.
Dagmar Kainmüller looks at countless photographs of this so-called sea snow. It is not always possible to make out anything, but her work is nonetheless incredibly important. The computer scientist and head of the Integrative Imaging Data Sciences working group at the Max Delbrück Centre for Molecular Medicine in the Helmholtz Association wants to use her view of a wide variety of plankton species to help other researchers find out more about the biodiversity of our oceans, nutrient cycles and, above all, carbon dynamics in the sea. This is in order to make our climate models even better. She is part of an interdisciplinary team of AI experts and marine scientists. The researchers plan to use the data to train a foundation model.
Dagmar Kainmüller. Picture: Pablo Castagnola/MDC
The term ‘foundation model’ is currently haunting the scientific community with as much promise as the hype surrounding ChatGPT around the world. The world-famous natural language processing application, which is used millions of times a day, is also a foundation model. But the Helmholtz Association is interested in more than just a text-based question-answering machine. Foundation models are designed to link large amounts of data and recognize correlations or patterns, so that important scientific questions can instantly be solved.
To do this, a foundation model is trained in several stages. First, it is fed with large amounts of well-prepared scientific data. Initially without a specific task. The aim is for the system to build up an extremely powerful knowledge base (the foundation) on its own. In the next phase, it can then be trained with relatively little effort to perform specific tasks, known as downstream tasks, which is what science is looking for. "With regard to plankton research, we hope to use the knowledge gained to better understand the nutrient and carbon dynamics of the oceans and thus optimize our climate models," says Kainmüller.
A potential basic model for plankton data which has not yet been developed would be fed with several billion photos that four Helmholtz Centers continuously generate in their research projects. Several hundred thousand of these images have already been annotated and categorized by scientists. This is known as annotated or labelled data.
For example, parts of the image are cut out of some of the original photos for basic training. The model learns to fill in the missing parts. Once the system is able to perform this task after countless repetitions, it is trained on various downstream tasks, such as identifying and visually highlighting different plankton species, distinguishing them from other species and organisms, or recognizing how much carbon is contained in the flakes, or how quickly the sea snow sinks to the bottom.
To accomplish this task, the trained basic model is augmented by an additional layer with, for example, just a few parameters. In contrast to basic training, the training input for this comes mainly from annotated data. The output of the model is then successively compared with the labelled initial data and refined until, in the best case, the result and the original input are identical.
Foundation models have enormous potential in all areas of research. In medicine, some systems are already established in everyday medical practice. Experts at the Centre for Medical Image Computing at University College London and the London NIHR Biomedical Research Centre at Moorfields Eye Hospital NHS Foundation Trust trained the RETFound model using 1.6 million retinal images. It is now able to diagnose diseases that manifest in the eye but whose symptoms occur elsewhere in the body, such as the risk of heart failure or heart attack.
The Helmholtz Association also wants to develop such models: From science for science, says Florian Grötsch, Senior Officer for Information & Data Science. The Helmholtz Association has everything it needs to ensure that developments in the field of AI for science and research are not left to commercial players alone: The data, the specialist knowledge of the researchers, the expertise in the field of artificial intelligence, sufficient computing power and the experience of our computer experts.
AI applications need a lot of computing power. With its booster module, the Jülich supercomputer JUWELS offers Germany's most powerful platform for AI. Picture: Forschungszentrum Jülich
To this end, the Helmholtz Foundation Model Initiative (HFMI) was launched at the beginning of February. Over the next three years, three pilot projects are to be set up using existing technology to create foundation models that offer clear added value for science and society. The application phase is currently underway. "The initiative is deliberately not specialized in one type of data," says Kainmüller, a member of the HFMI's four-person coordination committee. The decision on which projects to fund will be made at the end of May. The final results will be made available to the entire scientific community as open source projects: from the code to the training data to the trained models.
As with any algorithm and any AI, the consistently high quality of the training data is critical to the quality of the Foundation Models' results. Everyone involved in HFMI is aware of this challenge. As a result, special attention is paid to bringing the data sets up to the same quality standard. This is a huge undertaking, but it is the only way to realize the experts' hope that future models will better exploit the potential of existing data sets.
Another focus of the Helmholtz Association work on foundation models is to demystify the approach of artificial intelligence. "We know that the processes and workflows of AI are incredibly complex and difficult, if not impossible, to understand. There is a lot of data involved and no one can explain exactly how the result comes about. We want to understand this black box as best we can. We want to know what the model has learned," says Kainmüller.
"We are still doing basic and methodological research, and there are still many unanswered questions," adds Grötsch. How can the training data be curated so that the system produces the best results? Which parameters are relevant for the best result? How can I reliably sort out unusable data, such as image data? And at what point is the knowledge base good enough to stop training? One of the great promises of foundation models is the generation of synthetic data, i.e., the ability to replace time-, resource- and machine-intensive experiments with simulated data. At present, synthetic data still raises many questions, and it will be some time before foundation models can replace experiments, says Grötsch.
The number questions that need to be answered in order to gain a deep understanding of foundation models are increasing, not decreasing, every day. But that, says Kainmüller, is what makes this field of research so incredibly exciting. As exciting as snowfall deep below the surface of the water.
Readers comments