Deep Learning
Machines – and how they make decisions
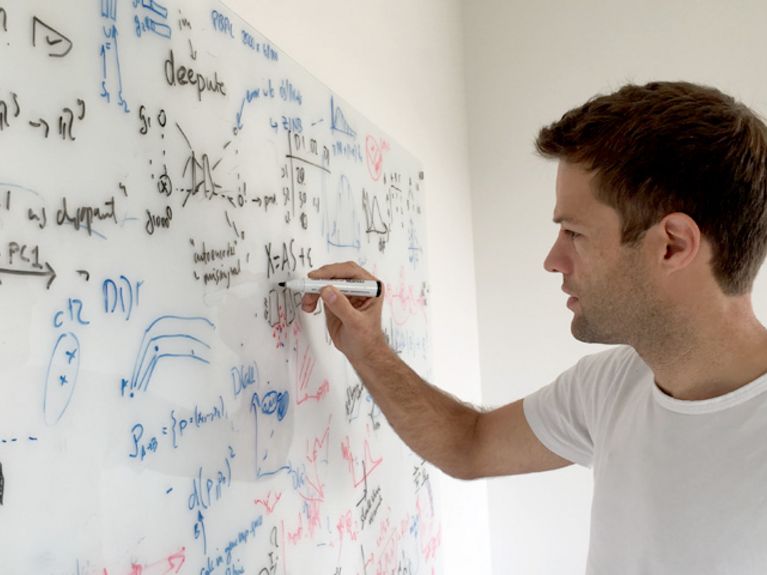
Prof. Fabian Theis from the Helmholtz Zentrum München “juggles” formulas. Picture: Helmholtz Zentrum München / Dr. Anna Sacher
Helmholtz researcher Fabian Theis teaches machines to learn so they can support the work of doctors and scientists. Theis spoke about the benefits offered by big data and machine learning at the Symposium for Individualized Infection Medicine in Hanover, Germany.
Laboratory technologies in the life sciences have progressed rapidly in recent years. A process that took an entire decade and consumed enormous resources in the 1990s – the sequencing of the human genome – is now carried out thousands of times each day in labs around the world. Gene transcripts are likewise sequenced on a routine basis. These short-lived copies of genes carry the blueprint for the proteins that a cell is producing at the time. Analyzing the transcriptome, which is the set of all the transcripts, provides researchers with information on the current condition of a cell, a tissue, or even an entire organism. Micro-scale methods have now developed to the point that the transcriptome or characteristics of individual cells can be examined in detail. This enables researchers to characterize different types of cells, their stages of development, or the ways they react to medications, for example.
However, these analyses create vast quantities of data – a phenomenon also referred to as big data. In addition to almost limitless series of genetic sequences, big data can also include other measured values or microscopic images. But most biologists and medical experts are not specialists in statistics or computer science. In other words, they need support to manage this flood of data.
Interpreting big data
One person they can turn to for this support is Fabian Theis, Director of the Institute for Computational Biology at the Helmholtz Zentrum München. “Big data doesn’t just mean large quantities of data, of course. The term also indicates that the data is complex and heterogeneous, and that it would be virtually impossible to interpret without the help of computers,” Theis explains. And while this data presents a challenge to many researchers in the life sciences, it is a big advantage for Theis’ research in the truest sense of the word: The more data he has access to, the more precise his results.
Theis holds a doctorate in physics and computer science, and one thing about him stands out in particular: He is incredibly enthusiastic about his work. During his lectures, he juggles figures and formulas that would make the average person’s head spin. And expert audiences, whether at Harvard University in the U.S. or in Hanover, Germany, value his expertise very highly. This is because Theis and his team have already developed numerous methods that make it possible to efficiently trawl through mountains of data in search of the latest findings.
His colleague Niklas Köhler, for example, is working on methods for searching through thousands of medical images of the ocular fundus for signs of diseased retinal tissue in order to prevent patients from losing their sight. And Alexander Wolf is mapping the development of stem cells in an organism with the help of big data analytics.
Teaching machines to learn
Theis carries out his work using machine learning, a method applied in the field of artificial intelligence. While computer programs that enable machines to learn from data have existed since the 1960s, a type of algorithm known as artificial neural networks has seen a revival in recent years. Thanks to the increase in their computing power, modern computers can work with software that is significantly more complex. Today’s neural networks sort and categorize characteristics on a number of hierarchical levels and “learn” from their experiences. This method is referred to as "deep learning" due to the numerous levels of learning involved. Deep learning enables neural networks to independently grasp the concepts underlying biological processes, for example.
Readers comments